In recent times, Artificial Intelligence has grown astronomically in how businesses, governments, and individuals conduct work.
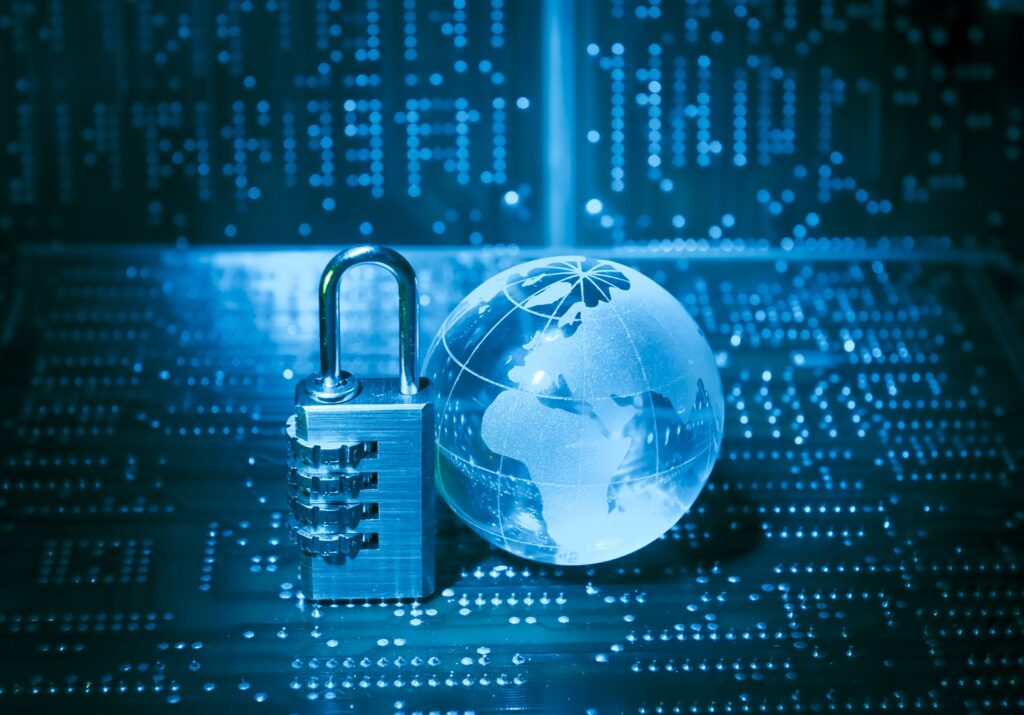
From recommendations to advanced robotics, industries have furthered the adoption of AI by bringing convenience, efficiency, and innovation into the mainstream.
However, in this rapid growth toward AI adoption, there is very often a hinterland of sensitive personal or organizational information data on which it depends. Given the rate at which cyber threats and data breaches are becoming an increasing occurrence in the modern digital environment.
Protecting data has never before been more critical. Protecting data maintains not only regulatory compliance under GDPR and CCPA but also the trust between the organization and the user community.
Understanding Data Protection
In contemporary society, characterized by digital trends, personal information protection has gained the limelight as one of the most important trust and compliance issues.
Data protection refers to practices and regulations put in place to protect individuals’ information from unauthorized access, misuse, or theft. It ensures that organizations responsibly handle data to preserve privacy and security.
Basic Principles of Data Protection
Such regulations may establish core data protection frameworks, such as the General Data Protection Regulation (GDPR) in the EU and the California Consumer Privacy Act (CCPA) in the US, based on the following core principles:
- Transparency: All entities should clearly inform individuals how information is to be collected and used.
- Data Minimization: Data shall be collected and processed only to the extent required for a specified purpose.
- Accountability: Organizations must use data in accordance with data protection legislation and demonstrate compliance.
- Individual Rights: Every consumer has the right to access, rectify, erase, or restrict the processing of their personal data.
These principles need to be center-stage in balancing innovation with individual privacy, especially in human-centered AI systems.
The Role of Personal Data in AI-Driven Systems
AI systems are data-driven; often, they rely on volumes of personal information to learn, improve, and offer accurate results. Examples include:
- Training Models: Most applications, such as personalized recommendations and predictive analytics, require personal data to develop algorithms.
- Enhanced Service Delivery: AI uses data to learn user behavior and improve the service experience.
Data Breach and Misuse Risks
This is not without some serious risks associated with the increasing use of personal data in AI:
- Data Breaches: Cyberattacks may lead to breaches resulting in identity theft, financial loss, and reputational damage.
- Privacy Violations: Improper handling could lead to violations of individuals’ privacy rights, causing distrust.
- Bias in AI: Poorly managed information may result in biased AI systems that discriminate against or are unfair to certain groups.
Opportunities: How AI Enhances Data Protection
Artificial Intelligence (AI) has certainly emerged as a transformative tool for enhancing data protection, offering innovative solutions to secure sensitive information in a world that’s increasingly digital.
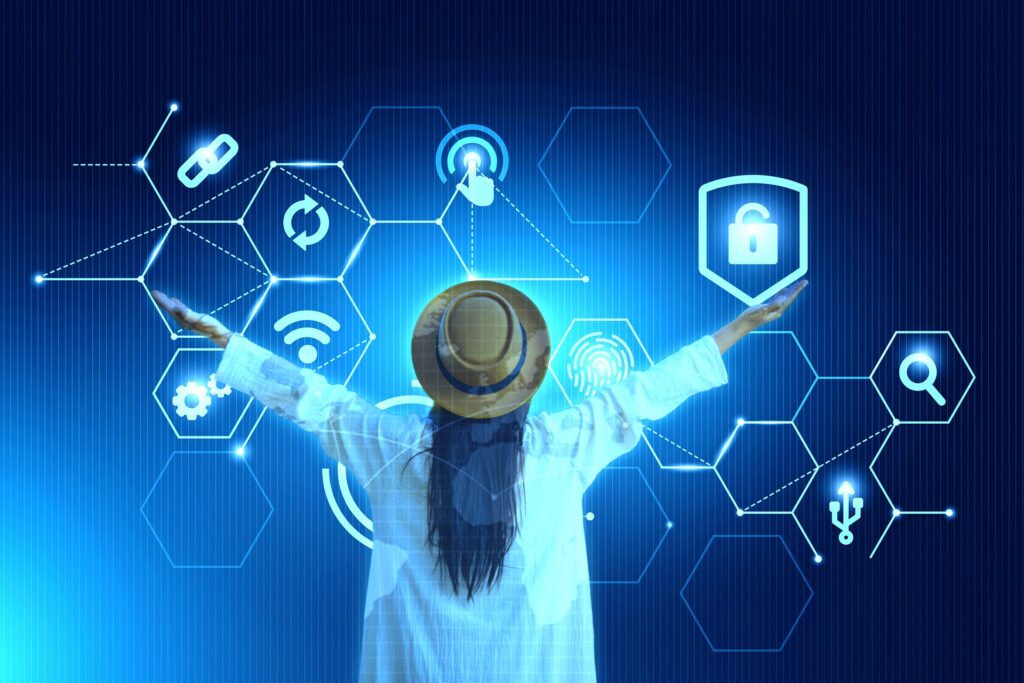
Real-Time Threat Detection and Response
AI systems analyze massive datasets to identify unusual patterns or threats. Unlike traditional methods, these systems operate continuously, enabling real-time detection and response to cyberattacks.
For instance, AI can flag suspicious logins, detect malware, or identify data exfiltration attempts before significant damage occurs.
Advanced Data Encryption
AI revolutionizes encryption by developing more secure methods to protect data during transmission and storage.
It optimizes encryption keys to resist brute-force attacks and uses machine learning models to anticipate and counter new hacking methods, ensuring robust data security.
Automating Compliance and Risk Management
Navigating regulations like GDPR or CCPA can be complex. AI simplifies this by automating audits, monitoring data use, and detecting regulatory violations.
It also generates reports to help organizations align with legal requirements while minimizing human error.
User Authentication and Access Control
AI enhances identity verification and access management systems through technologies like facial recognition, behavioral biometrics, and AI-driven multi-factor authentication, ensuring that only authorized individuals can access sensitive data.
Proactive Vulnerability Management
AI predicts system vulnerabilities before exploitation occurs. By analyzing historical data and attack patterns, it recommends patches or updates to secure systems, preventing breaches preemptively.
Data Masking and Anonymization
AI protects sensitive information by masking and anonymizing data, making it unidentifiable. This allows organizations to use data for analytics without compromising privacy, a critical need in industries like healthcare and finance.
Challenges of AI in data protection
While AI brings significant advancements, it also introduces challenges to data protection.
Data Privacy Risks
- Massive Data Reliance: AI requires extensive datasets, potentially leading to over-collection of information and privacy risks.
- Unintentional Exposure: Poor security practices or processing flaws can inadvertently expose sensitive data, compromising trust.
Bias and Ethical Concerns
- Biased Models: Incomplete or skewed datasets can amplify societal biases, leading to unfair outcomes in areas like hiring or credit scoring.
- Ethical Dilemmas: Balancing innovation with privacy can result in compromises, such as prioritizing business growth over data safeguards.
Transparency and Accountability
- Opaque Decision-Making: AI’s complex algorithms often function as “black boxes,“ making their outputs difficult to trace or explain.
Compliance Challenges: Auditing AI systems for regulatory adherence is time-consuming and technically demanding, complicating accountability.
Best Practices for AI and Data Protection
Adopting Privacy by Design
- Integrate privacy safeguards into AI systems from the start.
- Conduct risk assessments during development.
- Employ anonymization or encryption wherever possible.
- Implement default privacy settings that minimize data exposure.
Minimizing Data Usage
- Reduce reliance on personal data while maintaining model performance.
- Use federated learning to train AI across devices without transferring raw data.
Regulatory Alignment
- Ensure AI systems comply with evolving data protection laws like GDPR and CCPA.
- Stay updated on global regulations.
- Conduct regular audits to verify compliance.
- Support user rights, such as access, modification, or deletion of personal data.
Ethical AI Frameworks
- Build transparency and fairness into AI operations to foster trust and minimize bias.
- Design explainable AI models.
- Use diverse datasets to ensure equitable treatment across demographic groups.
- Continuously monitor and retrain AI to uphold ethical standards.
By adopting these measures, organizations can harness AI’s full potential while mitigating risks and fostering trust.
Case Studies: Balancing AI Innovation and Data Protection
As the organization embraces AI in driving innovation, responsible use to protect sensitive data is paramount. Notable success and failure examples that have made a balance in providing valuable lessons to others are as follows.
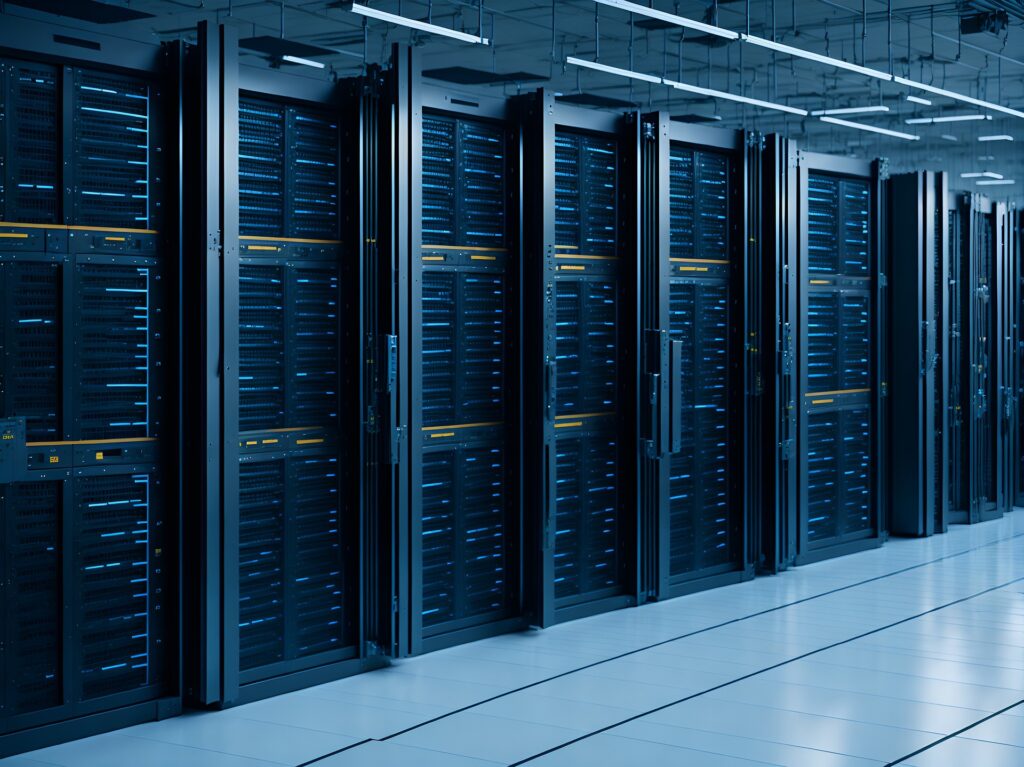
Success Stories: Building the Future with AI-Driven Data Protection
Google’s Implementation of Differential Privacy
Indeed, Google has been at the vanguard regarding the implementation of differential privacy a technique that allows no dataset to be reverse-engineered while preserving the utility of the datasets.
By doing so, Google can assess user behavior trends without having their personal data exposed, hence setting a gold standard for ethical AI applications.
Apple’s Machine Learning On-Device
Apple has made user privacy foremost, with on-device machine learning features like Face ID and Siri suggestions. In so doing, it restricts the volume of sensitive data that needs to be processed on cloud servers, reducing associated risks of data breaches and aligning with consumer expectations focused on privacy.
Mastercard Fraud Detection System
AI-powered algorithms run in real time to detect fraudulent transactions and protect billions of financial transactions happening globally with Mastercard. The manner in which anonymized data is used ensures that it has adhered to the protection of such data while guaranteeing a strong security structure.
Lessons from Legendary Failures
Cambridge Analytica Scandal
The Cambridge Analytica heist of Facebook data exposed the platform’s vulnerability to unauthorized data harvesting for AI-powered political profiling. It is reported that over 87 million users‘ data had been compromised in the breach, amidst public outrage and regulatory investigations.
Equifax Data Breach
Poor cybersecurity measures in Equifax in 2017 led to a data breach comprising sensitive information of 147 million. This data breach underlined how poor data protection can come forward in magnifying risks in AI models that are centric around data on consumers.
Legal Challenges Faced by Clearview AI
Clearview AI was accused of scraping billions of images from the internet without consent to build its facial recognition tool and became highly condemned internationally. It then faced several lawsuits and bans from a number of other countries.
Key Takeaways
- Success comes from innovation, implemented in parallel with ethical practices, such as adopting privacy-preserving technologies and decentralizing the processing of sensitive data.
- Failures underline the populace need for robust governance, transparency, and security measures.
The Future of AI and Data Protection
AI can greatly help in driving both the security and efficiency of data usage, yet at the same time, it poses some singular challenges.
Emerging Technologies: Safeguarding Data in New Ways
Differential Privacy
Differential privacy completely changes the ways in which an organization handles sensitive data. It will allow AI to determine trends in data without disclosing individual facts a way to maintain anonymity.
In fact, technology giants like Apple and Google have already begun using differential privacy so that usage statistics can be collected without compromising the privacy of a user.
Quantum Encryption
Quantum encryption will change the game in data security. Traditional encryption methods rely on mathematical formulae for protection, while quantum encryption relies on the principles of quantum mechanics to create virtually unbreakable security.
This may just be what is needed to ward off breaches even as computing power increases, making for robust protection of sensitive AI data sets.
Evolving Regulations: Driving Accountability
Global Standards for Data Protection
Laws like the EU’s GDPR, California’s CCPA, and India’s DPDP Act are making organizations rethink how AI works with personal information. These regulations call out transparency, data minimization, and user rightsall of which put stricter constraints on the proverbial box that houses an AI system.
AI-Specific Policies on the Horizon
The governments of the world are not keeping pace with drafting AI-specific regulations. The EU AI Act is one glaring example of categorizing AI applications based on risks and imposing strict requirements on high-risk systems.
Implementation of such policies will pave the way to align the development of AI with ethical and data protection standards.
The Role of AI in Data Protection
Proactive Threat Detection
AI will thus become a very critical tool in this process of trying to predict and hopefully prevent cyberattacks. Future AI systems, operating in real time with very large data sets, may find the existence of vulnerabilities before breaches can occur.
Better User Control
With regulations that want to bring transparency in place, AI systems will be giving the reins back to the users for controlling data.
Think of a world where intuitive dashboards lead the way and the daily user is in control of their data permissions and usage information.
Increased Collaboration Between Technology and Regulators
In balancing innovation and privacy, collaborations will be strengthened between developers of AI, governments, and data protection authorities. This synergy will drive the creation of ethical, secure AI solutions.
Conclusion
AI changes how we address data protection, providing at the same time powerful tools to protect sensitive information and unique challenges. It can’t be overemphasized: as organizations are leveraging AI for efficiency and innovation, it’s crucially important that safe data privacy is ensured.
Today, it is very much about the right balance between leveraging these capabilities of AI and robust adherence to a good standard of data protection principles. Conjectured ways to diminish these risks are through the implementation of privacy-by-design, data minimization, and keeping oneself updated with evolving regulations. In this regard, transparency and an ethical framework form the grounds on which responsibility and confidence in AI systems can be viewed.
Finally, the future of AI and data protection will be collaborative—between technology developers, businesses, regulators, and wider society. We can make this a landscape where AI provides security while not at the cost of privacy by working together. Innovation would then be able to embrace protecting the privacy of individuals.